This website uses cookies so that we can provide you with the best user experience possible. Cookie information is stored in your browser and performs functions such as recognising you when you return to our website and helping our team to understand which sections of the website you find most interesting and useful.
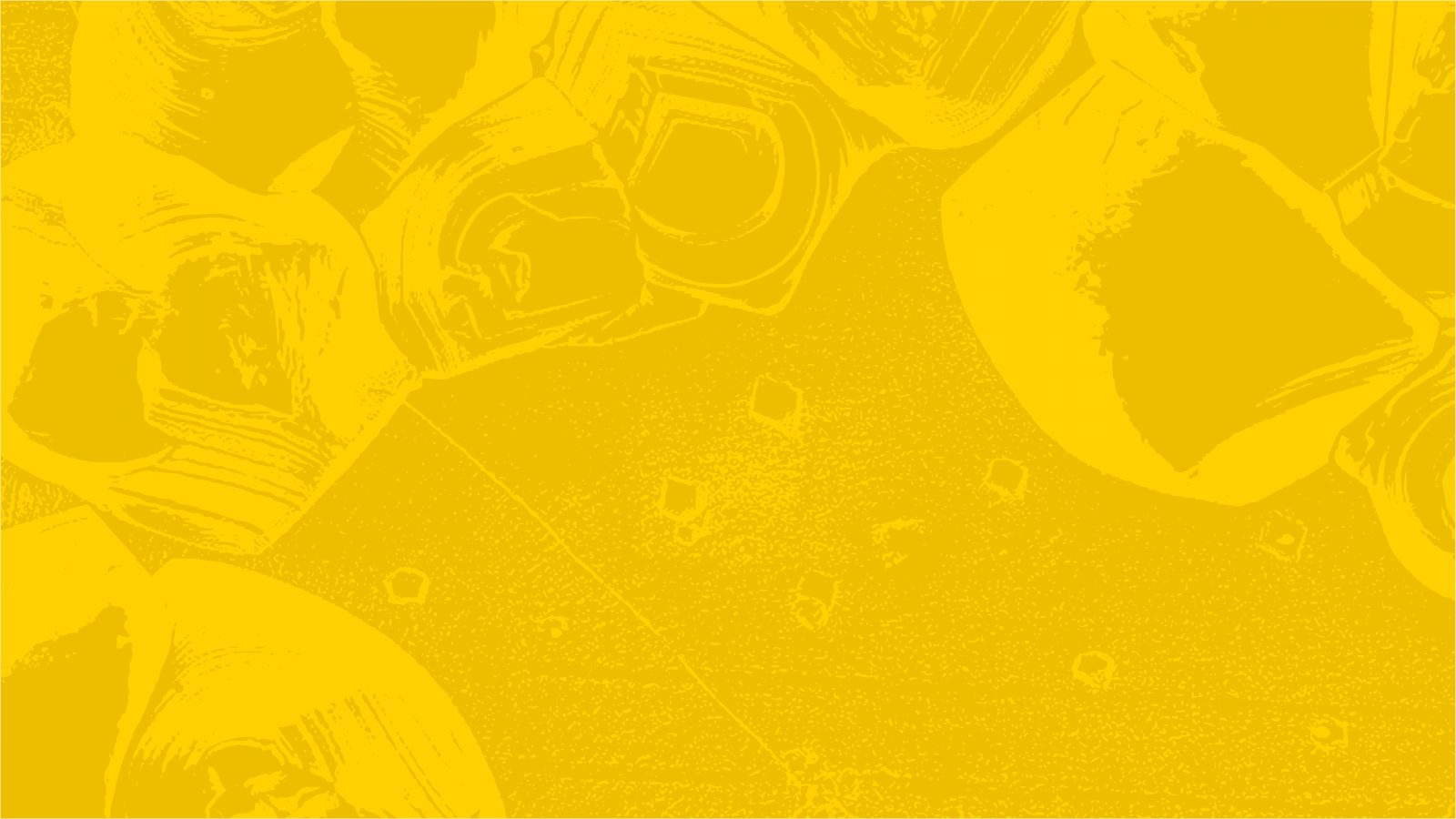
Developing Improved Aluminium Scrap Classification for Efficient Recycling
Case Study
Overview
The mechanical properties of aluminium alloys differ greatly across various grades. As a result, sorted aluminium scraps (according to grades), which can be recycled more efficiently for specific purposes, can yield considerably higher value compared to the unsorted ones.
In this work, the University of Warwick, collaborating with Total Metal Recovery Ltd, applied computer-vision-based methods to classify the grade of commercial aluminium scraps, for both pre-shredded and shredded scraps. To conduct this study, image datasets of both pre-shredded and shredded scraps were carefully curated, with each image containing a single scrap. Furthermore, a dataset comprising images featuring multiple mixed-grade shredded scraps was collected, and the potential of applying computer vision-based object detection was studied. Different neural network structures, including VGG16, Vision Transformers and Residual Neural Networks (ResNet) were tested on the curated data.
The experimental results and the feature analysis show that the computer vision-based methods can classify the shredded scraps with a promising high accuracy of 98.4%, while difficulty was observed in distinguishing pre-shredded scraps. By plotting the attention layers of the Vision Transformer and the class activation maps for the VGG16 and Resnet, we have visualised the features extracted by the neural networks. By analysing the extracted features, it shows that the neural networks focus on the edge and texture information of the scraps, potentially using the tracks left by the shredding process and material usage for classification.
About the Project
The aluminium industry is an essential component of the modern UK economy. Currently, the UK has only one primary aluminium smelter, producing approximately 50,000 tons annually. In contrast, in 2019 alone, the UK recycled 800,000 tons of aluminium, including 150,000 tons of high-purity scrap imported for specific applications, while exporting 450,000 tons of scrap to regions outside Europe. Recycling potential in the UK is forecast to reach an impressive 1.6 million tons per year by 2030. Recycling aluminium scrap can conserve up to 95% of the energy required for primary production. However, there is a notable disparity in the value of sorted and unsorted scraps.
Unsorted, mixed scrap fetches merely 15% of the price of the alloys that can be produced from it, while properly sorted aluminium can command 50 to 60% of the alloy price. To bolster aluminium recycling in the UK, new technology-enabled sorting will facilitate the production of high-quality aluminium alloys in a cost-effective, environmentally friendly manner. This study aimed to investigate the feasibility to conduct computer vision-based scrap classification for both shredded and un-shredded scraps. As a data-centric approach, the project’s cornerstone lies in the curation of dedicated scrap datasets for model training and testing.
The University of Warwick’s collaboration with Total Metal Recovery Ltd. facilitated the collection of samples and images representing commercial aluminium scraps, spanning various grades and in both shredded and un-shredded forms. For shredded scraps, we collected 1000 images for each of the 1000, 3000, 5000 and 6000 series. Each image captures a single scrap piece, under controlled conditions: fixed tripod-mounted camera, consistent lighting, and clean backgrounds. However, due to logistical constraints, acquiring a large quantity of un-shredded scraps was impractical. Consequently, images were captured at the scrap yard, resulting in backgrounds with more noise compared to the shredded samples. We obtained 900 images across the 3000, 5000, and 6000 series.
With the carefully curated dataset, extensive experiments were conducted to evaluate the performance of various neural network models on the classification task. Three models— VGG16, Residual Neural Networks (ResNet), and Vision Transformer (ViT), were trained on both shredded and un-shredded aluminium scrap datasets. ViT emerged as the most accurate, achieving 98.4% accuracy in shredded scrap classification. However, none of the models yielded satisfactory results in un-shredded scrap classification, with ViT achieving the highest accuracy at 61.0%. This highlights the greater variability in appearances of un-shredded scraps, suggesting that more samples are required to enable neural networks to learn features.
In addition to classification, we analysed feature and class activation maps for single and multiple scrap images. Feature maps revealed neural networks’ focus on edge and surface texture, potentially leveraging traces from shredding and material usage for classification. The class activation maps may hint towards the whereabouts of the scraps but exhibited limitations in accurately segmenting overlapping scraps. Nonetheless, these findings underscore the promise of neural networks in shredded scrap classification and suggest the potential for future research in object detection for aluminium scrap sorting.
This study explores computer vision’s potential in classifying aluminium scraps (shredded and un-shredded) by grades. Collaborating with Total Metal Recovery Ltd., diverse samples (varying grades of aluminium scraps) were collected. 1000 images for each grade of shredded scraps were obtained under controlled conditions, while 900 images for un-shredded scraps were captured in the scrap yard. The experimental results show that the computer vision-based method can be used for shredded aluminium scrap classification by grades. In addition, the potential of applying object detection for aluminium scrap sorting is examined with a dataset consisting of images having multiple scraps per image constructed, which can be used for future work.
Zushu Li
Professor, WMG, University of warwick
Collaborators
The work was completed with lead organisation University of Warwick, working with Total Metal Recovery Ltd.
The work was enabled due to a grant from the Henry Royce Institute’s Industrial Collaboration Programme.